As businesses experience rapid changes in the realm of supply chain management, staying ahead of the curve is essential. One critical aspect that can make or break a company’s success is demand forecasting and replenishment. Accurately predicting customer demand and efficiently managing inventory levels are vital for streamlining operations, reducing costs, and delivering exceptional customer service.
Artificial Intelligence (AI) has emerged as a game-changer in demand forecasting and replenishment. Utilizing machine learning (ML) techniques, AI enables businesses to make data-driven decisions, gain valuable insights, and optimize their supply chain operations. AI algorithms can analyze vast amounts of historical data, identify patterns, and generate accurate demand forecasts with a higher degree of precision than traditional methods.
In this article, we will explore the transformative power of AI-driven demand forecasting and replenishment and demonstrate how Algomine’s expertise and knowledge can help businesses achieve supply chain excellence. We will delve deeper into the concepts of demand forecasting and replenishment, explore the AI techniques that revolutionize these processes, and highlight the benefits and real-world examples of AI-driven success. We will also provide insights into how AI enables Logistics Managers, and Demand Planners, and discuss implementation process for AI-driven demand planning and replenishment. By the end of this article, you will have a comprehensive understanding of the role AI plays in optimizing demand forecasting and replenishment, and how our company can support your journey towards supply chain excellence.
We will also explore how to approach such a project, highlighting solutions for common challenges like managing slow-moving, new, or perishable products. Additionally, we will showcase the integration of popular tools like Databricks or SAS Viya with Power BI reports and business user interfaces. At Algomine, we are committed to delivering professional solutions while minimizing the need for costly software investments. Rather, we aim to use readily available resources to offer our clients customized solutions that meet their specific needs.
By implementing AI-driven demand forecasting and replenishment solutions, managers can achieve:
- Improved Inventory Management – Accurate forecasts enable better inventory optimization, reducing carrying costs while minimizing the risk of stockouts or overstocks.
- Elimination of Manual Inefficiencies – Streamlined replenishment processes paired with automated order generation contribute to more efficient operations.
- Increased Customer Satisfaction – By aligning inventory levels with customer demand, businesses can ensure timely product availability, enhancing customer satisfaction and loyalty.
Our deep understanding of demand forecasting methodologies, cutting-edge AI technologies, and industry best practices enables us to provide the state-of-the-art solutions our clients need. We are committed to partnering with businesses to transform their supply chain operations, drive growth, and achieve sustainable success.
UNDERSTANDING DEMAND FORECASTING AND REPLENISHMENT
Demand forecasting is the process of estimating future customer demand for a product or service. It involves analyzing historical data, market trends, and other relevant factors to predict the quantity and timing of future sales. Accurate results enable businesses to make informed decisions about production, inventory management, and resource allocation. It helps prevent stockouts, minimize excess inventory, and ensure optimal utilization of resources.
Replenishment, on the other hand, refers to the process of restocking inventory to meet anticipated demand. It involves determining when and how much inventory should be replenished based on the forecasted demand and logistical constraints. Effective replenishment strategies ensure that products are available to customers when needed, while minimizing carrying costs and reducing the risk of stockouts.
Key Challenges in Traditional Demand Planning Approaches
Traditional demand planning approaches often rely on historical sales data and subjective judgment, which can lead to inaccuracies and inefficiencies. Some of the key challenges are:
- Lack of Granularity – Traditional methods may not capture demand variations at a granular level, such as by SKU, location, or customer segment. This can result in suboptimal inventory management and missed opportunities for targeted marketing and sales strategies.
- Missed External Factors – Traditional approaches often struggle to incorporate external factors that impact demand, such as competition, market trends, and economic indicators. Ignoring these factors can lead to inaccurate forecasts and missed sales opportunities.
- Manual, Time-Consuming Processes – Traditional planning processes typically involve manual data collection, analysis, and decision-making. These processes are time-consuming and prone to errors, limiting the ability to respond quickly to changing market conditions.
Benefits of AI-Driven Demand Forecasting and Replenishment
AI-driven solutions provide substantial benefits over traditional approaches, particularly in the areas of demand forecasting and replenishment. These solutions overcome mentioned challenges while offering the following advantages:
Better Forecast Accuracy
By analyzing extensive datasets and identifying complex patterns, AI models can generate more accurate demand forecasts. Incorporating both internal and external factors, these models offer a holistic view of demand drivers, leading to less forecasting errors.
Enhanced Agility
AI solutions enable businesses to respond quickly to changing market dynamics and customer demands. Automated analyses support proactive decision-making and rapid adjustments to production, inventory, and distribution plans.
Optimal Inventory Management
ML models provide insights into demand patterns, seasonality, and customer behavior. This ensures that the right products are available at the right time and in the right quantities, reducing stockouts, excess inventory, and associated costs.
Cost Savings
Automating data collection, analysis, and decision-making processes reduces manual efforts, minimizes errors, and frees up valuable resources. Additionally, optimized inventory management lowers carrying costs and improves cash flow.
Scalability
By eliminating the need for manual labor, AI-based solutions can easily scale up without the need for additional staff. When the operations grow, ML models are trained on more data and produce results.
AI TECHNIQUES REVOLUTIONIZED THESE PROCESSES
ML’s capability to make predictions stems from its adeptness at uncovering intricate patterns and relationships concealed within historical data. These models autonomously tweak their internal parameters to grasp the underlying patterns inherent in the information. Demand forecasting often necessitates navigating complex relationships between numerous variables, encompassing elements like seasonality, trends, external influencers, and more. ML models possess the capacity to capture and represent these intricate relationships, which is pivotal for generating accurate predictions.
AI consistently delivers better results than older methods. In terms of speed and reproducibility, they clearly surpass any manual or expert-based solutions – especially when the number of time series starts to grow. On the other hand, simple econometric techniques, which are often available in operational systems such as ERP, are unable to reproduce complex relationships in the data and lack accuracy.
There are several machine learning techniques available for demand forecasting tasks. Generally, they fall into two main categories, depending on whether a single time series or a large dataset comprising various time series is employed for demand forecasting.
Single Time Series Forecasting
In this category, only a single time series of historical demand data is used for forecasting. Models concentrate on capturing patterns, trends, and seasonality within this lone sequence. Historically, this has been the most widely used approach. Despite its limitations, the simplicity of this method makes it a valuable tool in certain scenarios. Most popular examples of this class of models include:
- Autoregressive Integrated Moving Average (ARIMA) is a time series forecasting model that captures temporal dependencies and seasonality in the data. It’s effective for univariate time series data and can be useful for short-term demand forecasting.
- Seasonal Autoregressive Integrated Moving-Average (SARIMA) is an extension of ARIMA that accounts for seasonality in the data. It’s suitable for time series with strong seasonal patterns.
- Exponential Smoothing Models, such as Simple Exponential Smoothing (SES) are well-suited for time series data with various levels of seasonality and trend.
- Regression models are a class of machine learning algorithms that attempt to discover relationships between the variable of interest (e.g., sales) and a number of exogenous variables (such as prices, marketing efforts, or competition). Their main advantage lies in their ability to incorporate multiple factors that affect the target variable. However, using them for forecasting requires reliable plans for the future regarding all variables included in the model.
- Prophet developed by Facebook is designed for forecasting time series data that may have missing values, outliers, and multiple seasonality components. It can handle holidays and special events effectively.
Multiple Time Series Forecasting
In this case, a large dataset containing multiple time series is used for forecasting. This dataset can include demand for different products, regions, or other categories. All the state-of-the-art solutions implemented across the industries belong to this group. Although technically those algorithms could be used to model a single time series, their main strength lies in the ability to process large amounts of data and use them to extract even very intricate patterns. Examples include:
- Random Forest is a type of ensemble method that constructs multiple decision trees and aggregates their predictions.
- Gradient Boosting algorithms like XGBoost, LightGBM, and CatBoost are powerful ensemble methods that can capture complex relationships in data. They’re often used for both time series forecasting and demand prediction tasks involving multiple variables.
- Neural Networks areseep learning models, including recurrent neural networks (RNNs) and Long Short-Term Memory (LSTM) networks, can capture intricate temporal dependencies in time series data. They’re particularly useful for long-term forecasting and when dealing with sequences of varying lengths.
The choice of model depends on factors such as the characteristics of the data (e.g., seasonality, trend, noise), the availability of features, the forecasting horizon (short-term vs. long-term), and the specific business context. It’s often beneficial to experiment with multiple models and evaluate their performance using appropriate metrics before selecting the most suitable model for demand forecasting.
Once the predictive models are ready, it’s time to utilize their results in various optimization models, designed to automate and improve performance. From assortment and allocation, to order generation and warehouse operations, optimization can be used to streamline processes and support or make autonomous decisions. Methods in this area vary from linear and non-linear, or integer mathematical programming to heuristics based on genetic or simulated annealing algorithms.
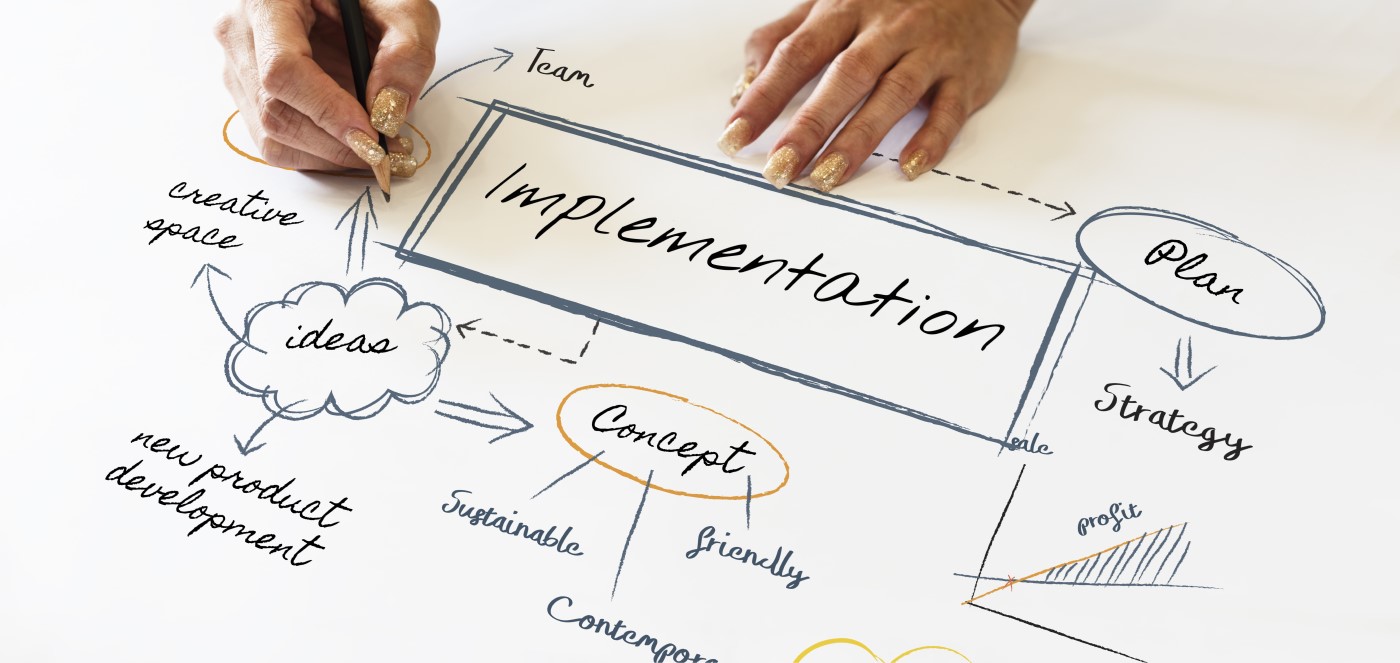
KEY ASPECTS OF SUCCESFULL IMPLEMENTATION
In our work, we’ve identified critical elements that are essential for successful implementation of projects, particularly those involving demand forecasting and AI technologies.
Data Availability
The quality and quantity of historical data directly influence the ability of forecasting models to capture underlying patterns, trends, and seasonality in demand. Adequate data enables models to learn from past behaviors and make informed predictions about future demand. Without a robust dataset, forecasting models may struggle to identify subtle variations, respond to seasonality, or account for external factors that impact demand. Additionally, accurate demand predictions often require diverse and comprehensive data sources that encompass customer preferences, market trends, economic indicators, and more. In essence, data availability is the foundation upon which successful demand forecasting strategies are built, as it empowers businesses to make well-informed decisions, optimize resource allocation, and ultimately improve overall operational efficiency.
Domain knowledge
Domain knowledge is a crucial asset in the implementation of machine learning models as it provides the context, insights, and understanding necessary to develop effective solutions. While machine learning algorithms excel at processing data and identifying patterns, domain knowledge bridges the gap between raw data and meaningful outcomes. It enables practitioners to select relevant features, interpret results, and make informed decisions that align with the unique nuances of a particular industry or field. Domain experts possess a deep understanding of the intricacies, challenges, and trends specific to their domain, allowing them to guide the formulation of hypotheses, the selection of appropriate variables, and the evaluation of model outputs for real-world relevance. In essence, domain knowledge enriches machine learning implementations by guiding model design, refining assumptions, and translating analytical outputs into actionable insights that resonate with the complexities of the given context.
Integration with business processes
The integration of machine learning models into business processes is pivotal for leveraging the full potential of data-driven insights. By seamlessly incorporating predictive analytics and forecasting into operational workflows, organizations can make informed decisions with greater accuracy and efficiency. Integrating ML models allows businesses to automate repetitive tasks, optimize resource allocation, and enhance strategic planning. This integration ensures that the insights generated by the models directly influence key areas such as inventory management, supply chain logistics, production planning, and marketing strategies. As a result, businesses can respond more effectively to changing market dynamics, minimize risks, seize opportunities, and gain a competitive edge. This process often requires an interface in which business users can display, review, or even correct and approve model results. Such solution makes it easier for business teams to utilize and connect with the ML solutions. Ultimately, integrating ML models into business processes not only improves decision-making but also fosters a culture of data-driven innovation, propelling organizations toward sustainable growth and success.
In other words, even the most accurate results will be useless unless the business puts them into service. This process requires trust in the solution or even dedicated training for business users. However, once the confidence is there, the organization can reap the full benefits of the implementation. Moreover, the feedback from business teams can be a valuable factor in further improvement of the models – they should continuously consult model results with the technical teams.
Continuous Learning and Model Refinement
One of the key advantages of AI in demand forecasting is its ability to continuously learn and adapt to changing market dynamics. As new data becomes available, AI models can be retrained and refined to improve their accuracy. This iterative process ensures that the models stay up to date and account for any shifts in customer behavior or market conditions. By continuously incorporating new data, businesses can make more accurate and timely demand forecasts, leading to better inventory management and operational decision-making.
In order to assure the quality of the models, it’s crucial to establish continuous monitoring of its results – such service should be an obligatory part of any deployment. This way, any sudden shifts in forecast quality can be easily and early detected.
Resources
Another important factor in assuring successful implementation of an AI solution is procuring necessary resources: both in terms of hardware and software (servers, licenses, libraries, cloud subscriptions) and competences (data scientists, ML and MOps engineers). Developing AI solutions at a scale might need various setups and can be resource-intensive, so its recommended to use expert advice about the shape and size of the required environment. Moreover, establishing an in-house data science competence center takes time – so if it’s an important factor, you might consider team augmentation or outsourcing services.
SOLUTION ARCHITECTURE
In data science projects, choosing the right architecture is crucial for ensuring the success and efficiency of the project. Different architectures offer unique advantages and cater to varying requirements. In this section, we concentrate on 3 popular approaches:
Cloud based solutions
Public cloud platforms such as Azure, AWS and have gained immense popularity in the data science community. Leveraging these cloud services provides scalability, flexibility, and easy access to a wide range of data processing and storage capabilities. Coupling the cloud platform with tools like Databricks make a perfect environments for data scientists to develop and deploy machine learning models. Additionally, integrating BI reporting tools allows for effective visualization and communication of insights derived from the data.
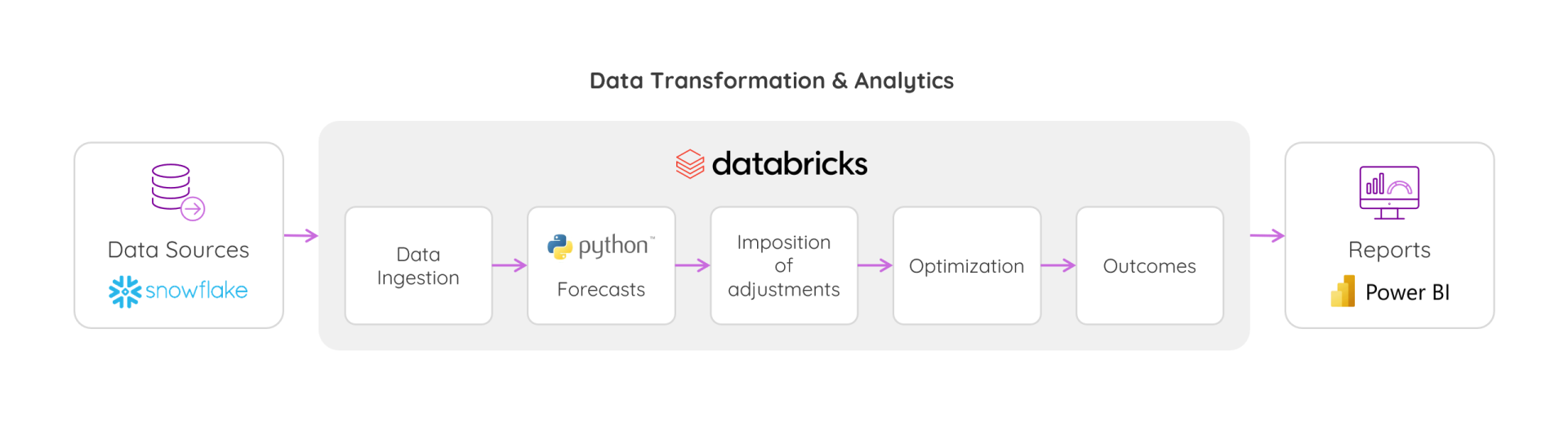
On-premise solutions
Some organizations prefer to keep their data infrastructure on-premises due to security, compliance, or specific business requirements. In this architecture, open-source coding languages like Python or R are commonly used for data processing and model development. On-premises solutions provide control over data privacy and security, allowing organizations to tailor their infrastructure to their unique needs. By incorporating BI reporting tools, insights can be shared effectively with stakeholders, enabling data-driven decision-making.
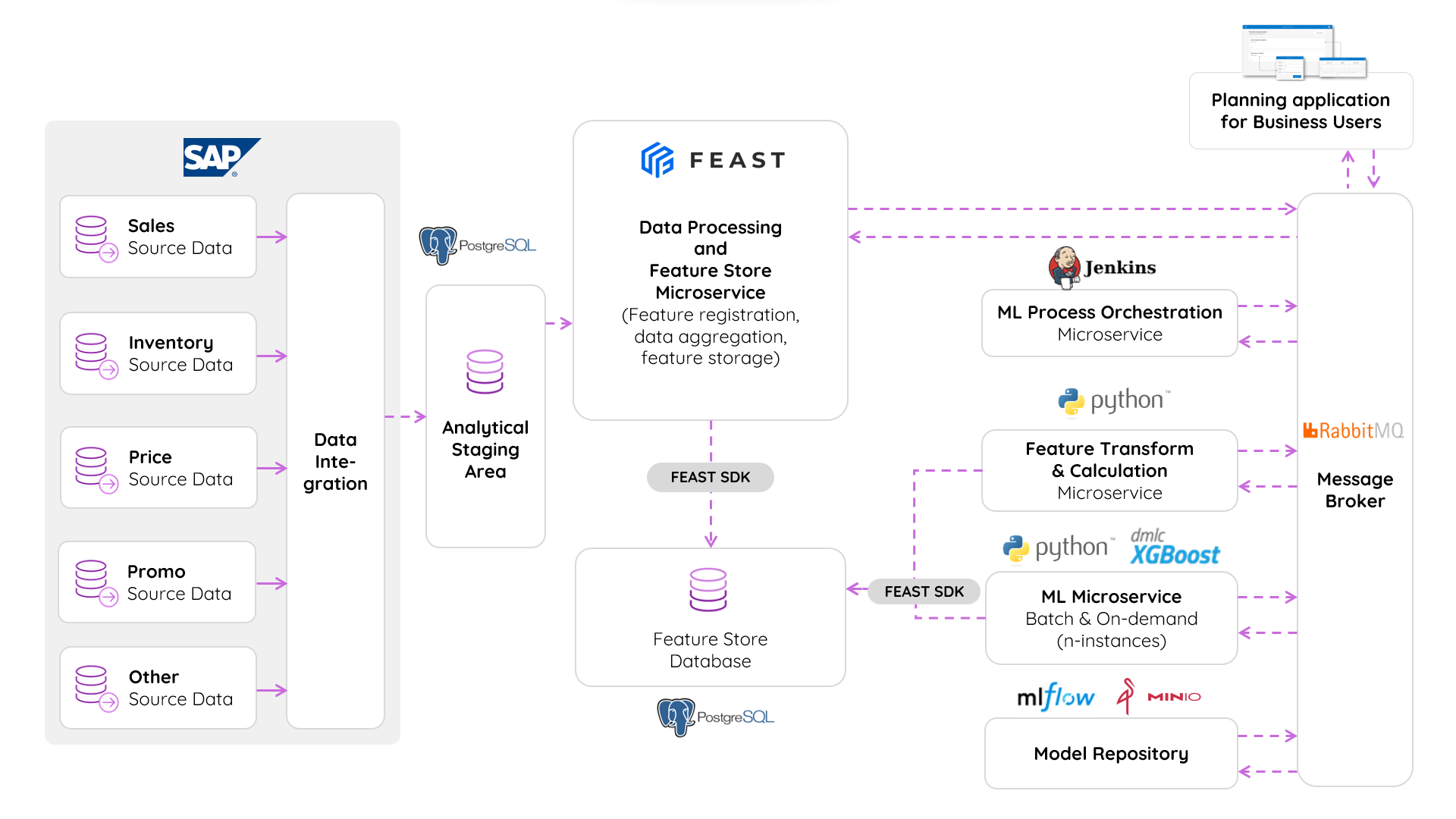
SAS solutions
SAS is a widely adopted software suite for data analytics and modeling. It offers a comprehensive set of tools and functionalities, including data management, advanced analytics, and reporting. It can be backed by on-premise servers, public and private clouds. SAS Visual Analytics enhances the capabilities by providing interactive and visually appealing dashboards, facilitating exploratory data analysis and intuitive reporting. This architecture is well-suited for organizations that have already invested in SAS and value its robust analytics capabilities, or require a support service provided by the company.
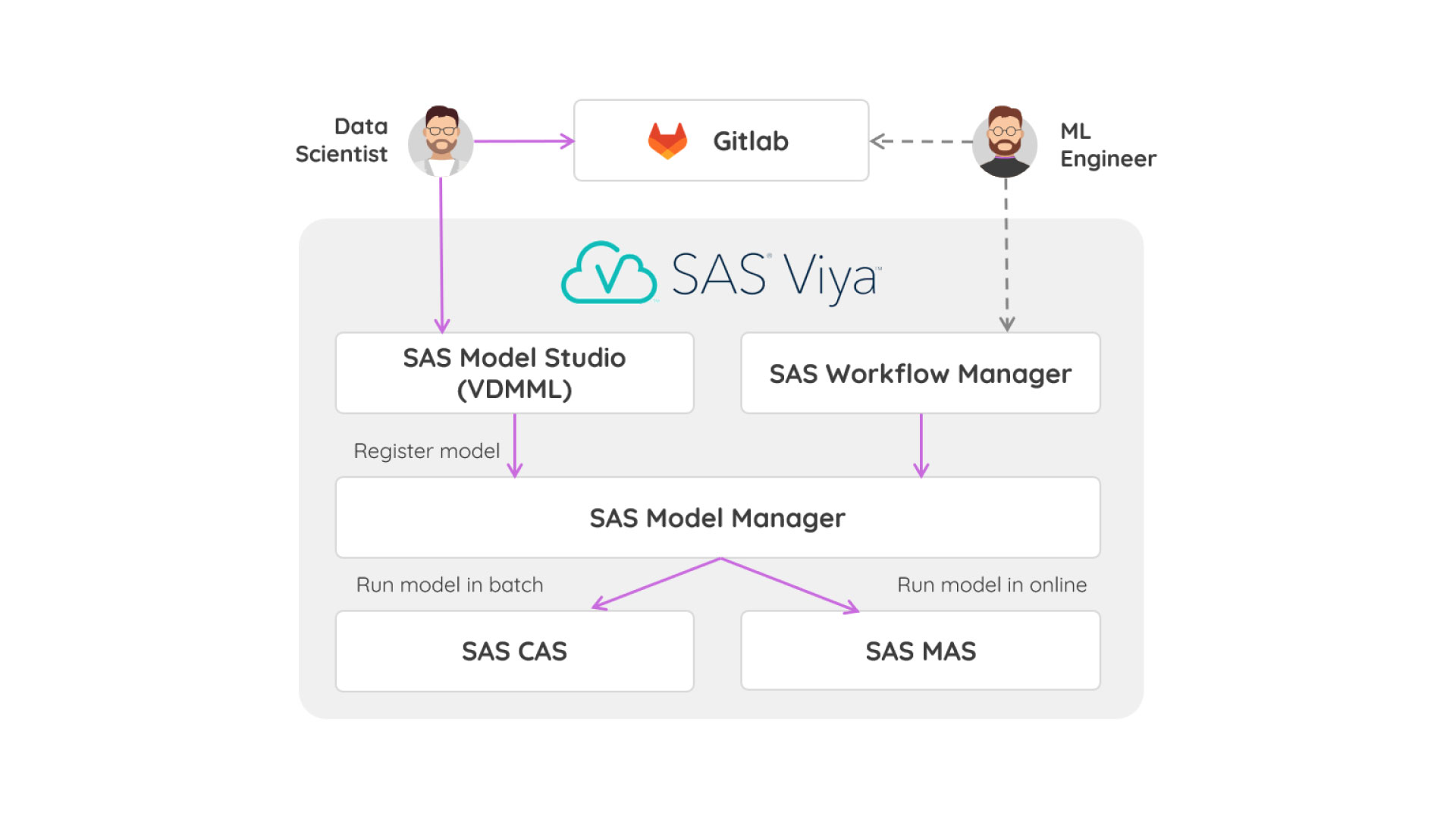
The demand planning application designed for business users
The demand planning application, designed specifically for business users, enables them to work effortlessly with forecast results generated by advanced machine learning models. They can analyze different scenarios and make purchasing decisions based on precise sales forecasts. If needed, users can independently apply expert adjustments, providing them with even greater flexibility in their actions.
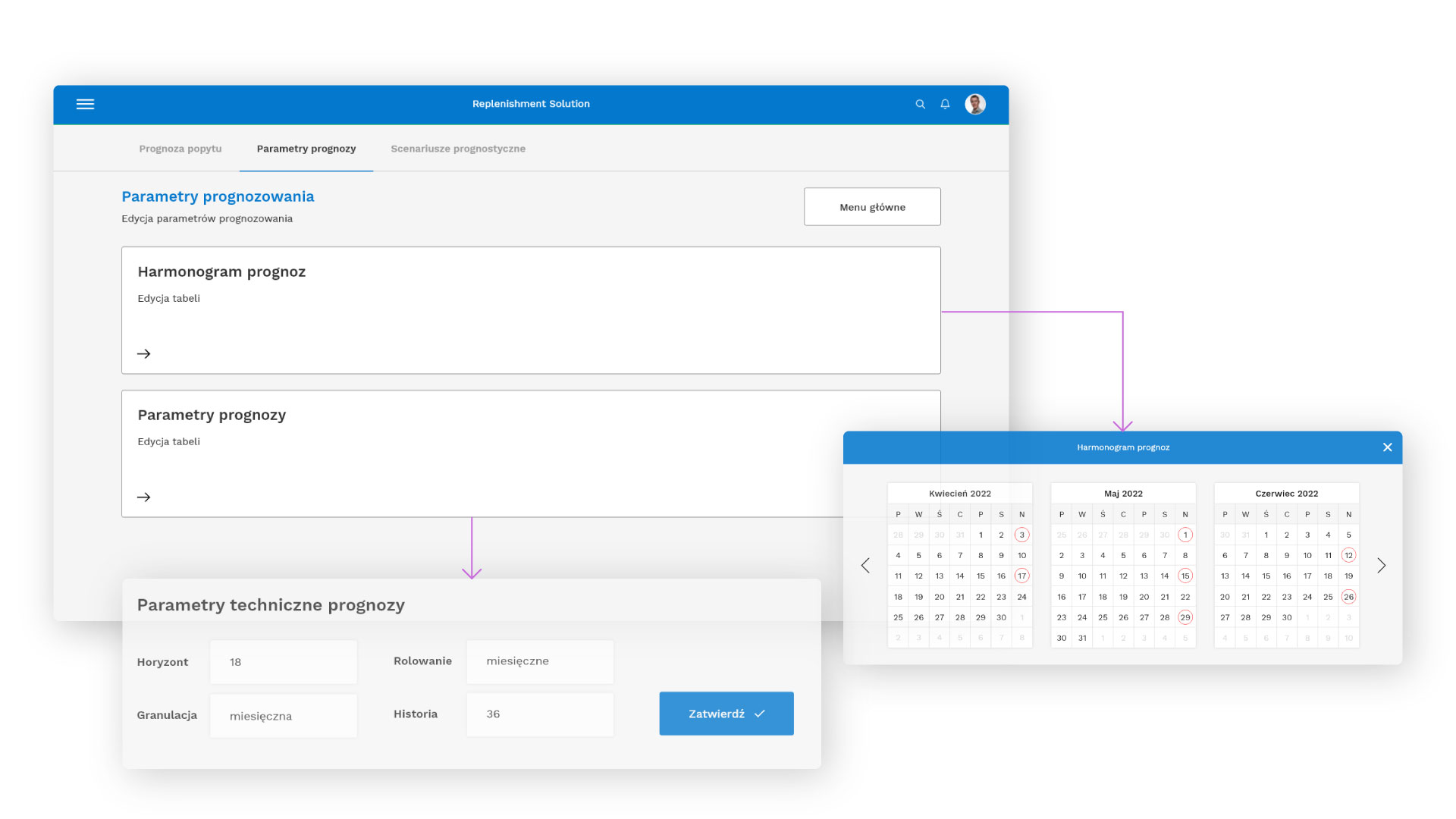
IMPLEMENTATION PROCESS AND TIMELINE
We understand the importance of a smooth transition and ensure that the implementation journey is well-planned and executed. Here is an overview of the typical implementation process and estimated timeline:
- Discovery and Assessment (2-4 weeks): Algomine’s team collaborates with key stakeholders to understand the unique requirements and challenges of the business. We conduct a thorough assessment of existing processes, data availability, and technology infrastructure to identify areas for improvement and tailor the solution accordingly.
- Data Collection and Preparation (2-4 weeks): High-quality data is essential for accurate demand forecasting. During this phase, we work closely with the client to collect historical sales data, customer behavior data, market data, and any other relevant information. We ensure data integrity, clean the data, and prepare it for analysis.
- Model Development and Training (4-6 weeks): Algomine’s team of data scientists and analysts develop advanced forecasting models using machine learning algorithms and statistical techniques. These models are trained on the prepared data to capture demand patterns, seasonality, and other factors influencing demand. We fine-tune the models to achieve optimal accuracy.
- Integration and Testing (2-4 weeks): Algomine’s solution is seamlessly integrated into the client’s existing systems and workflows. Initially the algorithm might work in the shadow mode, where results are produced but not yet visible in production. We conduct thorough testing to ensure data accuracy, model performance, and system compatibility. Any necessary adjustments or refinements are made during this phase to ensure a smooth integration.
- User Training and Knowledge Transfer (1-2 weeks): Algomine provides comprehensive user training to ensure that the client’s team is equipped to effectively utilize the solution. We offer guidance on interpreting forecast results, utilizing the replenishment recommendations, and leveraging the advanced analytics capabilities. Knowledge transfer sessions are conducted to ensure that the client’s team can independently manage and maintain the solution.
- Go-Live and Post-Implementation Support: Once the solution is implemented, Algomine provides ongoing support to address any issues or questions that may arise. We monitor the performance of the solution, conduct regular reviews, and provide recommendations for continuous improvement. Our team is readily available to assist with any queries or technical help
The duration of the implementation process may vary depending on the complexity of the business, data availability, and specific requirements. We have documented projects completed in 12 weeks, but large forecasting systems might require several months of development. Algomine takes a diligent and collaborative approach to ensure a successful implementation and a smooth transition to the new demand forecasting and replenishment solution.
REAL-WORLD EXAMPLES OF AI-DRIVEN SUCCESS
Electronics Retailer

The client, one of the largest retailers in the Polish market, aimed to improve the precision and automation of their sales forecasting process. They wanted to reduce instances of out-of-stock situations, optimize turnover of goods, streamline the planning process, and establish an advanced analytical platform.
Algomine’s approach consisted of three main components: developing an MLOps platform, implementing sales forecasting machine learning models, and creating a dedicated application for demand planning. The MLOps platform facilitated model deployment and maintenance, ensuring adherence to DevOps practices. The machine learning models were trained and deployed within the platform to generate sales forecasts. The planning application provided a user-friendly interface for analyzing forecasts and initiating appropriate actions.
The implementation involved integrating data from various sources into a staging database, preparing data tables in the feature store, and training models using the Gradient Boosting algorithm. The models considered historical sales, stock levels, pricing, promotions, shelf availability, and calendar events. The system was scalable, generating forecasts for tens of millions of time series.
Overall, Algomine’s approach to implementing MLOps and machine learning models resulted in improved sales forecasting capabilities for the client, enhancing their operational efficiency and decision-making processes.
Spare Parts Distributor
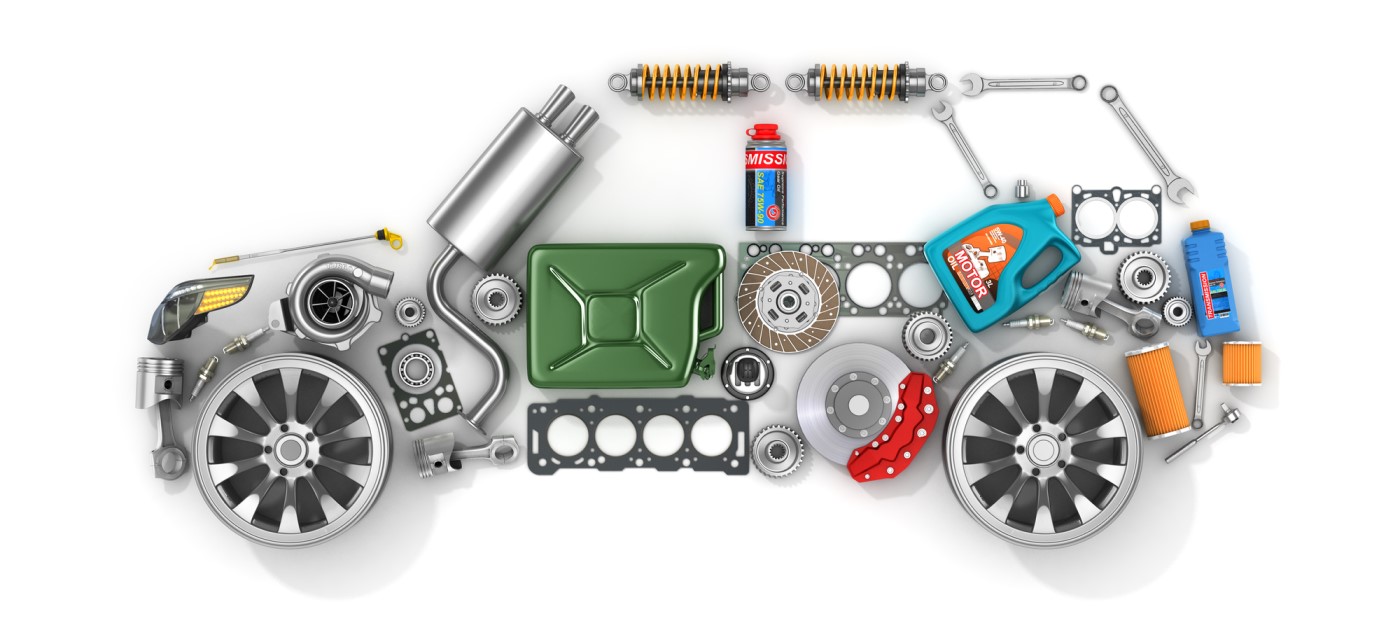
Our client, the largest distributor of automotive spare parts in central Europe, was encountering performance and accuracy issues with their previous forecasting solution. The results were calculated using simple statistical procedures and later manually verified by an expert. Due to the scale of their operations, both allocation and replenishment were fully automated – relying heavily on demand predictions. To address this challenge, we developed a custom algorithm for predicting low-frequency sales, forecasting over 8 million time series across 19 countries.
Our solution involved advanced data cleaning and augmentation processes, including anomaly detection for stockouts and outliers. Subsequently, we applied our customized approach to estimate seasonality patterns within the hierarchical structure levels. These patterns were then extrapolated to the time series with low-frequency sales. Finally, Machine Learning algorithms were employed to approximate long-term trends and incorporate weather effects into the forecasts.
As a result, our system enhanced forecast accuracy, thereby reducing instances of understocking and overstocking in the replenishment process. This led to fewer lost sales and improved profit margins. Additionally, the efficiency of calculations increased, reducing both time and resource consumption. Furthermore, skilled personnel were able to transition from manual oversight to more advanced tasks.
Pharmaceutical Manufacturer
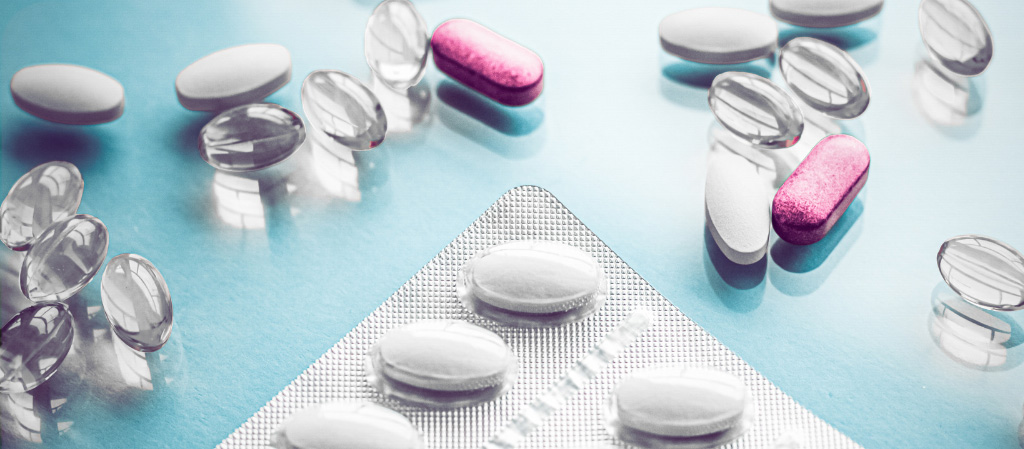
The client, a pharmaceutical company, faced challenges in accurately predicting sales and optimizing distribution across multiple products and regions. They sought to improve their forecasting accuracy to minimize stockouts and overstocks, enhance supply chain efficiency, and optimize resource allocation.
Our approach involved developing machine learning models to forecast sales and distribution. They collaborated closely with the client’s data engineers to integrate relevant data from various sources into a staging database. The data was then used to create data tables in a feature store, which contained the necessary business features for building the forecasting models.
The machine learning models were trained using historical sales data, product information, market dynamics, and other relevant variables. Algomine utilized advanced algorithms and techniques to capture seasonality, trends, and demand patterns specific to the pharmaceutical industry. The models were designed to handle the hierarchical structure of the data, considering different product categories and regional sales.
The implementation of the models allowed the client to generate accurate sales and distribution forecasts. These forecasts provided valuable insights for demand planning, inventory management, and resource allocation decisions. By optimizing their distribution processes based on the forecasts, the client was able to minimize stockouts, reduce inventory carrying costs, and improve customer satisfaction.
KEY TAKEAWAYS
- Algomine offers comprehensive AI-driven demand forecasting and replenishment solutions that address the unique challenges faced by businesses across industries.
- Our solutions leverage advanced technologies, such as machine learning and artificial intelligence, cloud-based technologies, expertise, pre-built assets, and commitment to provide accurate demand models and streamline replenishment processes.
- We believe in delivering professional solutions while minimizing the scale of necessary monetary investments. We leverage readily available cloud resources and utilize our existing assets to provide clients with tailored solutions that meet their specific requirements.
- Our implementation process is a well-structured journey that involves discovery, data preparation, model development, integration, training, and ongoing support.
- Well-executed implementation leads to improved forecast accuracy, enhanced agility and responsiveness, optimal inventory management, cost savings and efficiency, and scalability.
By partnering with Algomine, businesses can expect transformative changes in their demand forecasting and replenishment processes. Algomine’s deep industry expertise, proven track of records, and commitment to client success make us an ideal partner for businesses seeking robust and effective demand forecasting and replenishment solution