OUR CLIENT
For our client, the largest insurance organization in Poland, we developed and implemented a suite of advanced machine learning solutions to enhance the efficiency of automotive insurance claim resolutions.
These algorithms were integrated into an automated processing system to accurately route claims to the appropriate resolution paths and streamline their valuation. The result was a significant reduction in unnecessary handling costs and an improvement in customer satisfaction.
THe company, established in 1921, employs over 11,000 people across 400 locations and achieved a record revenue of 27 billion PLN in 2023.
BUSINESS CHALLENGE
Our client faced several key challenges:
- Efficiently processing a high volume of automotive insurance claims, each with numerous potential resolution paths.
- Developing an automated decision-making system capable of handling multiple decision points while providing customer service employees with accurate claim estimates.
- Ensuring high levels of customer satisfaction by optimizing processing times and delivering precise, reliable outcomes.
OUR APPROACH
To address these challenges, we helped our client automate its claim processing by employing advanced machine learning models to assign claims to the appropriate resolution paths. Specifically, we developed three key models to support decision-making:
- Damage Settlement Cost Estimation: This model predicted the final repair value by analyzing historical claims, repair bills, and customer data. Its outputs were used as the basis for initial offers and during settlement negotiations.
- Offer Acceptance Probability: This model assessed the likelihood of offer acceptance during settlement negotiations. It evaluated the risk associated with each claim by considering multiple variables, enabling more informed decision-making.
- Damage Evaluation Assignment: This model determined whether claims should be processed through automated (more efficient) or manual (more precise) damage evaluation paths, balancing efficiency and accuracy.
All three models were integrated into an online automated claim processing system, providing real-time decision support for customer service employees.
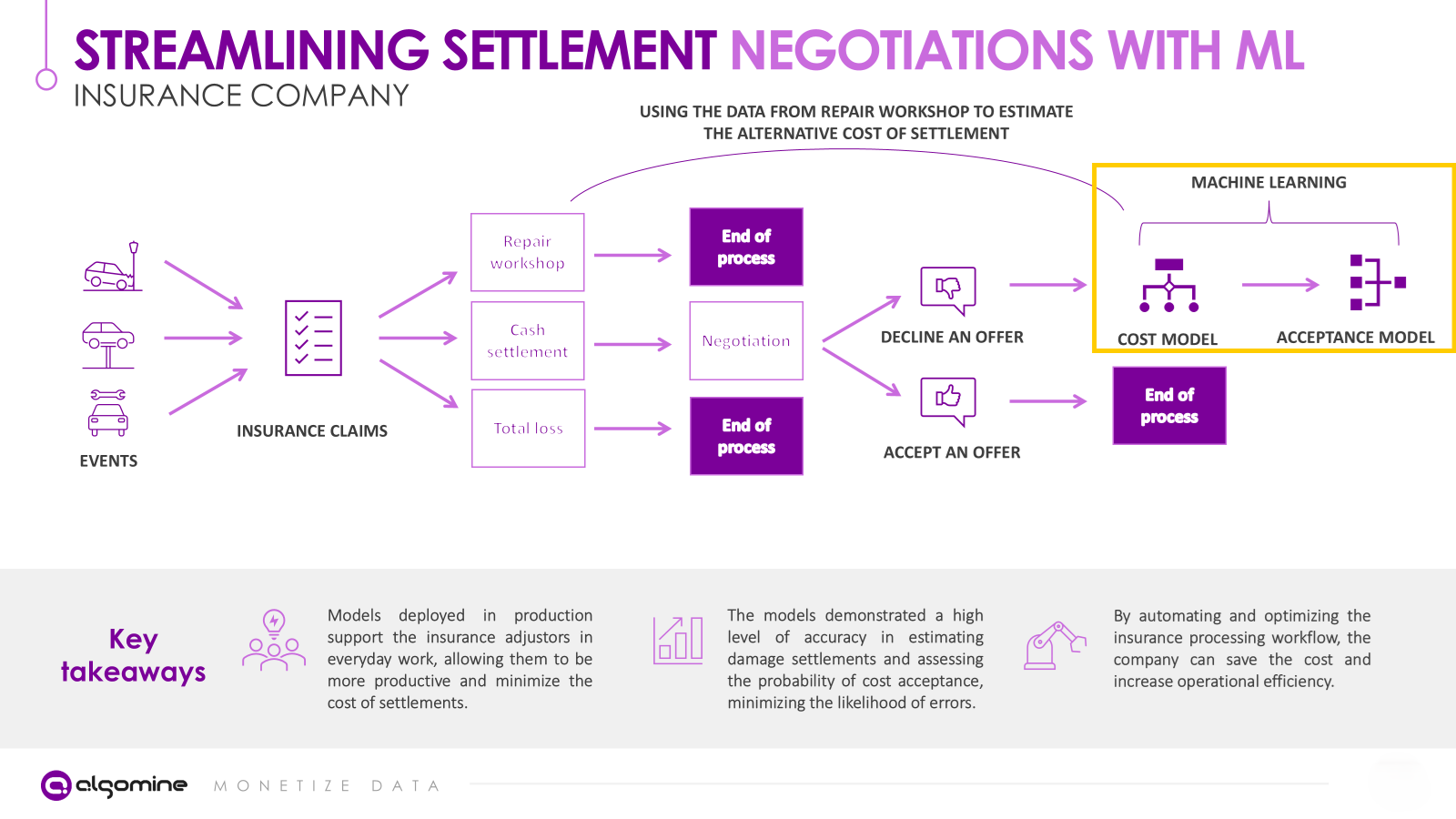
THE RESULTS
In high-volume insurance operations, even minor inefficiencies can result in significant unnecessary costs. By implementing machine learning models, our client was able to address this challenge, optimizing the assignment of claims to the most appropriate resolution paths and providing accurate settlement offers for each customer.
The insights generated by these models proved invaluable during settlement negotiations. Precise estimations of both the alternative repair costs and each customer’s likelihood of offer acceptance significantly reduced overall settlement costs. Additionally, the accurate allocation of technical experts to more complex evaluations lowered labor expenses while shortening resolution times, ultimately enhancing customer satisfaction.